Works presented at Congresses and Conferences
Download PdfVideo-Based Fruit Detection and Tracking for Apple Counting and Mapping
Gené-Mola J., Felip-Pomés M., Net-Barnes F., Morros J.R., Miranda J.C., Arnó J., Asín L., Lordan J., Ruiz-Hidalgo J., Gregorio E., 2023. Video-Based Fruit Detection and Tracking for Apple Counting and Mapping. Proceedings of the 2023 IEEE International Workshop on Metrology for Agriculture and Forestry (MetroAgriFor), Nov 6-8, 2023, Pisa (Italy). PP 301-306. ISBN 979-8-3503-1272-0/23
Automatic fruit counting systems have garnered interest from farmers and agronomists to monitor fruit production, predict yields in advance, and identify production variability across orchards. However, accurately counting fruits poses challenges, particularly due to occlusions. This study proposes a multi-view sensing approach using continuous motion videos captured by a camera moved along the row of trees, followed by fruit detection in all video frames and application of Multi-Object Tracking (MOT) algorithms to prevent double-counting. Three tracking methods, namely SORT, DeepSORT, and ByteTrack, are compared for fruit counting using the YOLOv5x object detector. The methodology is applied to map fruit production in an experimental apple orchard at two different dates: four weeks and one week before harvest. The results demonstrate that ByteTrack (MOTA = 0.682; IDF1 = 0.837; HOTA = 0.689) outperforms SORT and DeepSORT, indicating its superior tracking performance. Computational efficiency analysis reveals similar processing times between SORT and ByteTrack (about 15 ms), while DeepSORT requires significantly more processing time per image (128 ms). Fruit counting evaluation shows reasonably accurate yield predictions on both dates, with reduced errors and improved performance closer to the harvest date (MAPE = 7.47 %; R2 = 0.70). The system proves effective in estimating orchard fruit production using computer vision technology, offering valuable insights for yield forecasting. These findings contribute to optimizing fruit production and supporting precision agriculture practices. The code and the dataset have been made publicly available and a video visualization of results is accessible at http://www.grap.udl.cat/en/publications/video_fruit_counting.
Amodal segmentation for on-tree apple fruit size estimation with RGB-D images
Gené-Mola J., Ferrer-Ferrer M, Gregorio E., Blok P.M., Hemming J., Morros JR., Rosell-Polo JR., Vilaplana V., Ruiz-Hidalgo J. 2023. Amodal segmentation for on-tree apple fruit size estimation with RGB-D images. Anual Catalan Meeting on Computer Vision (ACMCV2023). Poster. https://repositori.udl.cat/handle/10459.1/463463
The detection and sizing of fruits with computer vision methods is of interest because it provides relevant information to improve the management of orchard farming. However, the presence of partially occluded fruits limits the performance of existing methods, making reliable fruit sizing a challenging task. While previous fruit segmentation works limit segmentation to the visible region of fruits (known as modal segmentation), in this work we propose an amodal segmentation algorithm to predict the complete shape, which includes its visible and occluded regions. CONCLUSIONS The main advantages of the present methodology are its robustness for measuring partially occluded fruits and the capability to determine the visibility percentage. Future works should evaluate the performance of the method with commercial RGB-D sensors, which would facilitate data collection.
A new Leafiness-LiDAR index to estimate light interception in intensive olive orchards
Sandonís-Pozo, l., Martínez-Casasnovas, J.A., Escolà, A., Rosell-Polo, J.R., Rufat, J., Pascual, M. 2023. A new Leafiness-LiDAR index to estimate light interception in intensive olive orchards. In: Staford, J (Ed.), Precision Agriculture'23, 14th European Conference on Precision Agriculture, Wageningen Academic Publixhers, Wageningen (The Netherlands), pp 189-195. DOI: 10.3920/978-90-8686-947-3, https://repositori.udl.cat/handle/10459.1/463686
Modern fruticulture is moving towards the adoption of new monitoring tools to support decision-making in orchard management practices. Particularly, in olive orchards, canopy light interception constitutes a key factor in yield and oil quality. A new index, named Leafiness-LiDAR index (LLI), is presented, which combines a geometric parameter (canopy cross-section) with a structural one (leafiness), both obtained from mobile terrestrial laser scanner (MTLS) derived 3D point clouds. Also, production and quality parameters were collected and analysed. To validate the results, the photosynthetically active radiation (PAR) was measured with a ceptometer, showing a significant correlation with the LLI (r = 0.8). The index also showed a significant relationship both with production parameters such as pruning percentage (r = 0.8) or olive production (r = 0.6) and with quality parameters: polyphenols content, oil stability and bitterness (r = 0.6). The results indicate that LLI may be useful to adopt early decisions on pruning or fertigation, as well as for variable input rate applications in the framework of Precision Fruticulture.
This work was carried out with data acquired in the project IRTA2012-00059-C02-01 funded by the Instituto Nacional de Investigación y Tecnología Agraria y Alimentaria, , as well as in the projects AGL2010-22304-C04-03 and AGL2013-48297-C2-2-R funded by the Spanish Ministry of Economy and Competitiveness and developed under the frame of the Grant RTI2018-094222-B-I00 (PAgFRUIT project) by MCIN/AEI/10.13039/501100011033 and by “ERDF, a way of making Europe”, by the European Union.
Uncertainty analysis of a LiDAR-based MTLS point cloud using a high-resolution ground-truth
Lavaquiol, B., Llorens, J., Sanz, R., Arnó, J., Escolà, A. 2023. Uncertainty analysis of a LiDAR-based MTLS point cloud using a high-resolution ground-truth. In: Canavari, M., Vitali, G., Mattetti, M. (Eds.), Book of Abstracts (Posters), 14th European Conference on Precision Agriculture, 2-6 July 2023, Bologna, Italy. pp 37. https://repositori.udl.cat/handle/10459.1/463872
The study of plant geometry is crucial to design specific management by providing the optimal quantities of nutrients, fertilizers, pesticides and irrigation rates. Before the advent of the first 3D characterization systems, it was very laborious to obtain accurate commercial scale 3D crop data. Nowadays, there are sensing systems which allow 3D canopy characterization to be performed in a relatively simple and fast way. LiDAR (light detection and ranging) sensors have been widely used in agriculture. When 3D scanning techniques are used, it is essential to be aware of the total measurement error. One of the limitations when using real data is the absence of ground-truth (GT) to compare the obtained measurements . In a previous research [1], validated a high-resolution 3D point cloud on an actual defoliated tree obtained from RGB images and stereo-photogrammetry techniques. This accurate 3D point cloud can be used as digital ground-truth (DGT) to validate 3D LiDAR point. The accuracy of the scanning system includes the errors committed by the sensor, the positioning system (GNSS), the data acquisition set up, the point cloud generation algorithms and the georeferentiation of the DGT.
Grants: Spanish Ministry of Science, Innovation and Universities through the projects PAgFRUIT (RTI2018-094222-B-I00) and PAgPROTECT (PID2021-126648OB-I00) (by MCIN/AEI/10.13039/501100011033 and by “ERDF, a way of making Europe”, by the European Union).
How can precision agriculture contribute to the 50 % pesticide reduction goal of the farm-to-fork strategy?
Llorens, J., Román, C., Escolà, A., Gené-Mola, J, Arnó, J., Martínez-Casasnovas, J.A. 2023. How can precision agriculture contribute to the 50 % pesticide reduction goal of the farm-to-fork strategy? In: Staford, J (Ed.), Precision Agriculture'23, 14th European Conference on Precision Agriculture, Wageningen Academic Publixhers, Wageningen (The Netherlands), pp 285-292. DOI: 10.3920/978-90-8686-947-3.
The Farm-to-Fork (F2F) strategy of the European Green Deal demands a 50 % reduction in the use of pesticides by 2030. In this work, several Precision Agriculture solutions were used in a vineyard spray application and the results were compared to a conventional treatment. Three different treatments were applied with the farm air-blast sprayer in a commercial 2.5 ha vineyard. Treatment 1 was the conventional farm application of a uniform 450 L ha-1 volume rate. In Treatment 2, the decision support system (DSS) DOSA3D was used to decide an adjusted uniform volume rate of 430 L ha-1. Treatment 3 took advantage of several PA techniques: a) electronic canopy characterization using a mobile terrestrial laser scanner to map canopy geometric parameters; b) management zones delineation; c) DSS to decide two different dose rates to create a prescription map (low vigour: 340 L ha-1 and high vigour: 430 L ha-1); d) variable-rate technology to execute the prescription map implemented in the farm sprayer. The spray deposit on leaves was the parameter used to assess differences between treatments. The statistical analysis did not show significant differences between treatments in high vigour vines. However, in low vigour vines, the mean deposits of both uniform treatments increased and showed significant differences with the variable-rate treatment.
Grants: Spanish Ministry of Science, Innovation and Universities through the projects PAgFRUIT (RTI2018-094222-B-I00) and PAgPROTECT (PID2021-126648OB-I00) (by MCIN/AEI/10.13039/501100011033 and by “ERDF, a way of making Europe”, by the European Union).
Low-cost terrestrial photogrammetry for orchard sidewards 3d reconstruction
Martínez-Casasnovas, J.A., Rosell-Tarragó, M., Rosell-Polo, J.R., Sanz, R., Gregorio, E., Gené-Mola, J., Llorens, J., Arnó, J., Escolà, A. 2023. Low-cost terrestrial photogrammetry for orchard sidewards 3d reconstruction. In: Canavari, M., Vitali, G., Mattetti, M. (Eds.), Book of Abstracts (Posters), 14th European Conference on Precision Agriculture, 2-6 July 2023, Bologna, Italy. pp 113. https://repositori.udl.cat/handle/10459.1/464591
Large-scale fruit tree canopy geometric and structural characterisation is of interest in Precision Fructiculture to assess spatial variability in orchards. Tree dimensions and structure and their temporal evolution is strongly connected to light interception and tree status and should be considered in management decision making processes. Aerial and terrestrial laser scanning is one of the usual solutions when it comes to canopy characterisation. However, results may be of poor resolution for the former and expensive to be adopted as commercial farm solutions for the latter. UAV-based photogrammetry is becoming popular but monitoring tall fruit tree crops (> 3 m) may result in a loss of canopy information such as shape and porosity. The authors present an alternative which consists in using terrestrial photogrammetry techniques traveling with a ground-based vehicle along the orchard alleyways to monitor canopies sidewards. In order to obtain sufficient image overlap in tall fruit tree orchards (> 3 m tall with between 4 m or 5 m row spacing), a vertical structure was designed to attach 3 sensors at different heights. The sensors used were the cameras of 3 mobile phones and an app was used to take pictures at a given frequency. The open-source computer program Meshroom 2021.1.0 (Alicevision) was used to reconstruct the scene and the resulting point clouds were compared to a reference point cloud obtained with a mobile terrestrial laser scanner. The average difference between both point clouds (only including points in a the tree row section of 7.5 m long and 2 m wide) was 0.019 m and ranged from 0 m to 0.811 m. The 96.5 % of the points presented differences less than 0.05 m. The system presents some flaws related to the acquisition rate, the image resolution, the number of images to process and the overall processing time. The first two could be solved by using other cameras and last two could be solved with the real-time reconstruction capabilities of some photogrammetry programs, to be explored in future works.
Grants: Spanish Ministry of Science, Innovation and Universities through the projects PAgFRUIT (RTI2018-094222-B-I00) and PAgPROTECT (PID2021-126648OB-I00) (by MCIN/AEI/10.13039/501100011033 and by “ERDF, a way of making Europe”, by the European Union).
Assessment of different N treatments in Hedgerow Almond Orchards by means of LiDAR point clouds
Sandonís-Pozo, L., Llorens, J., Escolà, A., Arnó, J., Pascual, M., Martínez-Casasnovas, J.A., 2022. Assessment of different N treatments in Hedgerow Almond Orchards by means of LiDAR point clouds. XXI International Nitrogen Workshop 2022. Madrid 24-28 Octubre 2022. http://hdl.handle.net/10459.1/84121
Monitoring of canopy status in fruit tree orchards allows better decisions in the canopy management, such as pruning or fertirrigation. LiDAR is an effective tool to acquire accurate 3D geometric and structural data, such as height, width, volume or canopy porosity, among others. In the present work, a super-intensive almond orchard (Prunus Dulcis) with 8 different N treatments (N50, N100, N150, NStop: N100 only in Fase I and with and without DMPSA nitrification inhibitor in 24 rows and 3 blocks, was scanned during three years (2019-21) in two different vegetative stages (after spring pruning and before harvesting) by means of a terrestrial LiDAR scanner. Canopy parameters such maximum height and width, cross section and porosity were summarized from the LiDAR 3D point cloud every 0.5 m along the almond tree hedgerows. A repeated measure mixed statistical model was applied to each parameter in order to assess the effect of the N treatments. The adjusted R2 ranged from 0.73 of the canopy width to 0.83 of the porosity. Canopy parameters and their main interactions with the different treatments were significantly differentiated. The N100+DMPSA treatment was the one favoring higher canopy development (higher cross sections and widths, and less porosity), while the NStop+DMPSA treatment was related to lower canopy development and higher porosity.
This work was carried out with data acquired in the project IRTI2018-094222-B-I00 (PAgFRUIT project) by MCIN/AEI/10.13039/501100011033 and by “ERDF, a way of making Europe”, by the European Union.
Gené-Mola, J., Sanz-Cortiella, R., Rosell-Polo, J.R., Escolà, A., Gregorio, E. 2022. Apple size estimation using photogrammetry-derived 3D point clouds. Anual Catalan Meeting on Computer Vision 2022, Universitat Autònoma de Barcelona, (http://acmcv.cat/). https://unidisc.csuc.cat/index.php/s/ARswqDsavb6VWRZ
Sandonís-Pozo, L., Plata-Moreno, J.M, Llorens, J., Escolà, A., Pascual Roca, M., Martínez-Casasnovas, J.A., 2022. PlanetScope Vegetation Indices to Estimate UAV and LiDAR-derived Canopy Parameters in a Super-Intensive Almond Orchard. Frutic 2022, Valencia, 29 June - 1 July 2022 (https://www.frutic2022.com/). Link to the presented paper HERE.
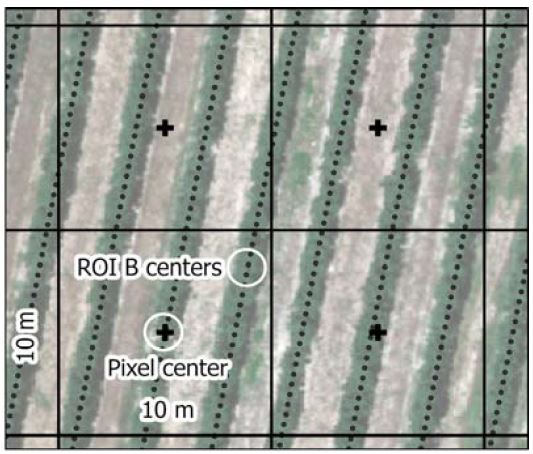
Martínez-Casasnovas, J.A., Llorens, J., Sandonís, L., Escolà, A., Arnó, J., 2021. NDVI from satellite images to estimate LiDAR-derived geometric and structural parameters in super-intensive almond orchards. In: Stafford, J.V. (ed.), Precision Agriculture’21. Wageningen Academic Publishers, Amsterdam (The Netherlands), pp 567-572. https://doi.org/10.3920/978-90-8686-916-9. PostPrint document.
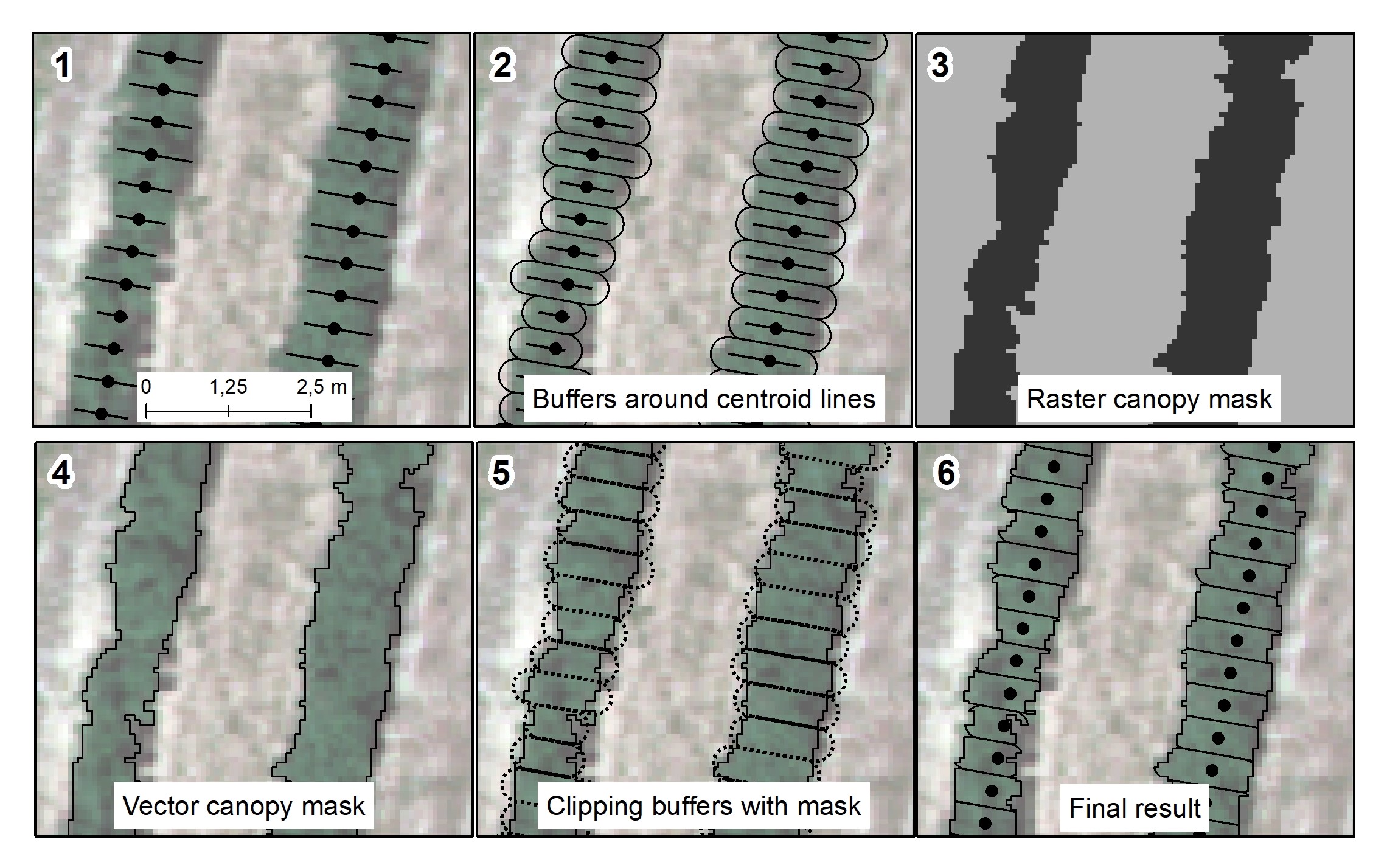
Llorens, J., Escolà, A., Rosell-Polo, J.R., Arnó, J., Martínez-Casasnovas, J.A., 2021. Estimation of geometric and structural parameters in a super-intensive almond (Prunus dulcis) orchard from multispectral vegetation indices derived from a UAV image. In: Stafford, J.V. (ed.), Precision Agriculture’21. Wageningen Academic Publishers, Amsterdam (The Netherlands), pp 129-135. https://doi.org/10.3920/978-90-8686-916-9. PostPrint document.
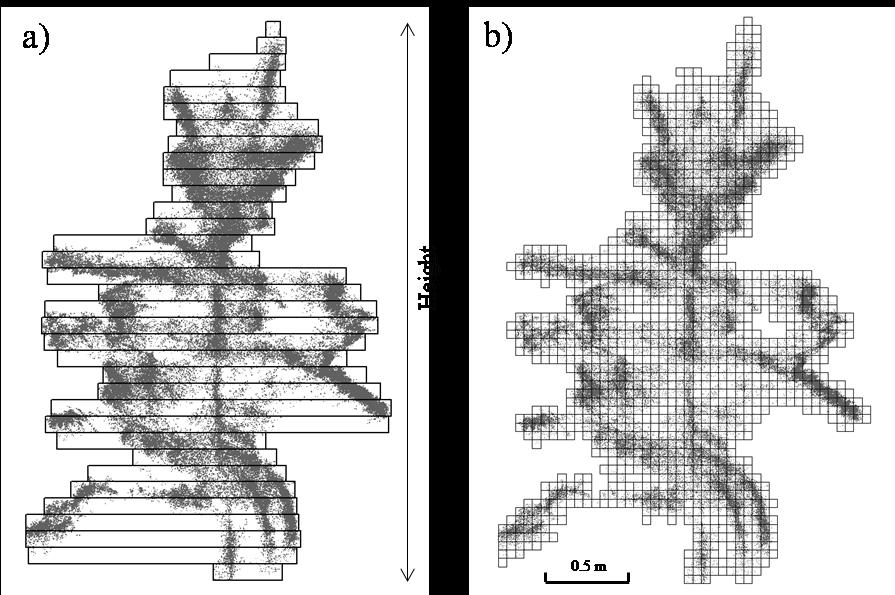
Llorens, J., Alsina, A., Arnó, J., Martínez-Casasnovas, J.A., Escolà, A., 2021. Multi-beam LiDAR-derived data analysis for optimal canopy 3D monitoring in super-intensive almond (Prunus dulcis) orchards. In: Stafford, J.V. (ed.), Precision Agriculture’21. Wageningen Academic Publishers, Amsterdam (The Netherlands), pp 395-401. https://doi.org/10.3920/978-90-8686-916-9. PostPrint document.
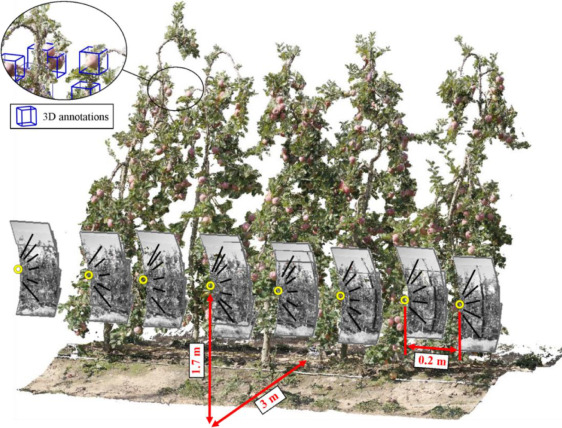
Gené-Mola, J., Sanz-Cortiella, R., Rosell-Polo, J.R., Morros, J.R., Ruiz-Hidalgo, J., Vilaplana, V., Gregorio, E., 2020. Fruit detection and 3D location using instance segmentation neural networks and structure-from-motion photogrammetry 7th Annual Catalan Meeting on Computer Vision, Barcelona, 22th September 2020. Link to the poster.